Faster, cheaper genome sequencing and more intelligent AI algorithms promise a new era of precision medicine.
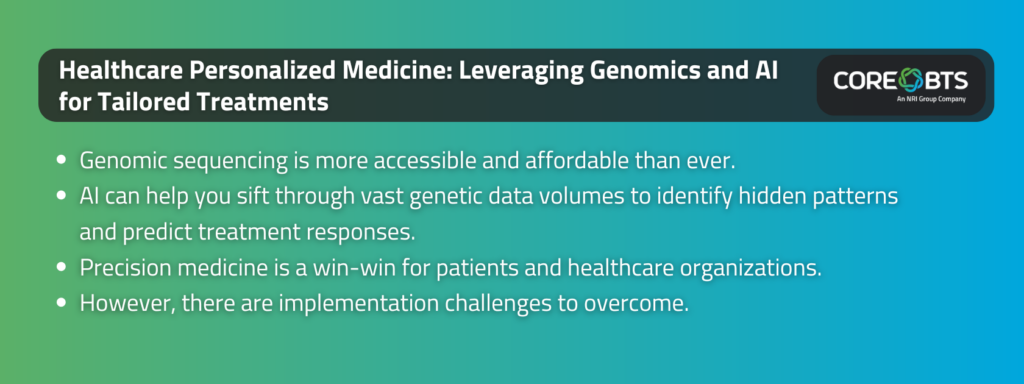
Genomics and artificial intelligence improve healthcare through cheaper, more effective, tailored treatments. This article explores the intersection between these two technologies and covers critical considerations when navigating this exciting new era of precision medicine.
Read on to discover how personalized healthcare boosts patient outcomes, contributes to your bottom line, and what the future holds.
Advancements in Genomics
Just 30 years ago, the prospect of whole genome sequencing (WGS)—determining the unique configuration of over 6 billion nucleotides in a genome—seemed impossible in terms of cost, time, and effort.
The Human Genome Project, which provided the first sequence and an invaluable human genome reference, took an astounding 13 years (October 1990 – April 2003), and its final costs reached $2.7 billion.
The project’s extraordinarily high cost was primarily due to reliance on the low-throughput Sanger method, which sequences one DNA fragment at a time.
Fast-forward to 2024, genomic sequencing has become more accessible and affordable. Improved methodologies like next-generation sequencing (NGS) have allowed for considerably higher throughput and efficiency. Unlike Sanger sequencing, the NGS method sequences millions of fragments in parallel, reducing WGS costs to as low as $200.
This incredible milestone facilitates the integration of genomic testing into clinical workflows, enabling detailed genetic profiling, earlier diagnoses, and personalized treatment plans for various diseases, including rare genetic disorders.
With artificial intelligence (AI) advancing rapidly, healthcare institutions have an unprecedented opportunity to harness genomic data for precision medicine.
Role of AI in Medicine
As genomic sequencing costs decrease, data volumes are increasing. According to the National Human Genome Research Institute, a single WGS exercise generates about 200 gigabytes of raw data.
AI can help glean meaningful insights from this avalanche of genomic data for better patient care. Artificial intelligence easily tackles tasks like genome annotation, variant calling and classification, and phenotype-to-genotype correspondence. These tasks often create errors when addressed using standard statistical procedures. Plus, they’re impractical to perform using human intelligence, making AI the logical choice.
Machine learning (ML) and deep learning (DL) are the most common forms of AI in clinical genomics.
Traditional ML involves supervision, meaning you provide the system with a well-defined training dataset (input) to learn from, which it then uses to make predictions about a separate test dataset (output). In contrast, DL is unsupervised, and algorithms independently recognize patterns in data and make predictions by mimicking how the human brain works.
These AI techniques are valuable in clinical genomics as they can find hidden correlations and determine the treatment protocols most likely to succeed based on treatment contexts and patients’ unique genetic attributes. A reputable healthcare IT consulting partner can help you leverage them effectively.
Developing Customized Treatments
Patients respond to treatments uniquely, highlighting the limitations of traditional symptom-driven approaches. Healthcare providers can harness genetic markers and other causal factors by integrating AI with genomics to create personalized, effective treatment plans. This innovative approach enhances treatment efficacy while reducing the risk of side effects, paving the way for a more tailored healthcare experience.
For example, studies show that Han Chinese patients with the HLA-B*1502 alleles are highly likely to develop toxic epidermal necrolysis and Stevens-Johnson syndrome from the seizure drug carbamazepine. However, these adverse side effects can also affect patients of different descents with HLA-B*1502 alleles.
AI can help you compare genetic markers against known side effects at scale before initiating therapy to prevent adverse outcomes. Similarly, it can help you make the best prescription based on how previous patients with similar conditions responded to treatment.
Benefits to Patients
Targeted therapies are more effective than standard “one-size-fits-all” treatments.
A good example is the trastuzumab and ado-trastuzumab emtansine (T-DM1) therapies used to treat HER2-positive breast cancer patients. These tailored cancer treatments boost patients’ survival rates by 90% and reduce the risk of recurrence by half compared to traditional chemotherapy.
Put differently, leveraging AI and Genomics to aid clinical diagnosis and treatment brings you closer to delivering the best patient outcomes.
Impact on Healthcare
Replacing trial-and-error prescribing with a scientific approach can help your organization minimize unnecessary procedures and readmission costs. Studies show these cost savings can sometimes be as high as 34%.
Knowing which patients will benefit from a treatment and what alternatives to pursue for their counterparts makes it easy to avoid wasting resources. AI and genomics make this possible through personalized medicine.
Challenges and Ethical Considerations
While AI is superhuman in identifying meaningful patterns in complex genomic data, it can sometimes lead to discriminatory, unethical, and even false conclusions.
For example, biased algorithms may predict greater disease likelihood based on race and gender when they aren’t causal factors. Or they may not provide accurate predictions for groups underrepresented in training data.
Similarly, AI systems may struggle to provide a justified explanation for predictions involving complex interdependencies, which can make it difficult to establish accountability around bias and errors they make.
And then there’s the issue of privacy: How do healthcare organizations source data for training AI systems and ensure bad actors can’t trace it back to patients during a breach?
These are all vital concerns, and healthcare organizations and regulators must establish robust AI governance mechanisms and frameworks to minimize negative impacts. AI is one of the most consequential and powerful technologies in healthcare, so it will require thoughtful policy and continuous attention for many years.
Future Directions
We expect many exciting genomics and AI developments in the years ahead. For example, the cost of whole genome sequencing will likely fall below $100 within the next decade, making it even more accessible and affordable.
At the same time, healthcare organizations will widely adopt AI in clinical practice. Generalized AI tools will become the standard in tasks requiring frequent inference from complex data, such as genome annotation and variant calling.
These AI tools will augment, not replace, human clinicians. So, healthcare organizations must communicate this point effectively and train their staff accordingly. Over time, human clinicians will move towards job designs and tasks that draw on uniquely human skills like persuasion, empathy, and big-picture integration.
Healthcare AI systems will likely be sufficiently standardized, integrated with EHR systems, and regulated within the next decade. Preparing for this new era of personalized medicine is paramount.
Leverage Genomics and AI for Personalized Healthcare With Core BTS
As the leading digital transformation partner of the world’s most trusted healthcare brands, Core BTS is on a mission to equip frontline clinicians with cutting-edge technology so that they never compromise on patient care. Healthcare is a hectic business, and we’re committed to helping you make it less so.
Talk to us about where you are on your journey toward embracing genomics and AI for personalized medicine. Let us help you chart a path toward strategic healthcare today and tomorrow. Contact us now to learn more.
Share on